Multiscale Modeling and Simulation
Multiscale modeling or multiscale mathematics is the field of solving problems that have important features at multiple scales of time and/or space.
In physics and chemistry, we use multiscale modeling to calculate material properties or system behavior on one level. To do this, we might use information or models from different levels. On each level, we use particular approaches to describe the system correctly.
Most materials have some complexity of structure at the nano or microscale that influences their behavior at the continuum level. Our goal is to build a valid continuum model that captures this complexity of the micro and mesoscale. To do this, we need to bridge the gap between molecular scale models and the continuum.
Understanding Continuum-level Material Behavior
Materials scientists have for many years tried to understand continuum-level material behavior by looking closely at the material’s nanostructure. For example, we might want to study structure and properties at this scale. In this case, we can use molecular and mesoscale dynamics simulations based on classical equations of motion. However, using the results directly within finite element models is not entirely straightforward.
The purpose of this blog is to describe a suitable workflow to:
- Bridge the gap between very fine-grained work at the atomic/molecular level
- Work through a mesoscale simulation level
- Understand continuum-level material behavior for use in finite element (FE) simulations of real components.
Figure 1. A multiscale workflow to derive material properties using multiscale molecular modeling tools in finite element methods and beyond
Bridging the Gap
There are many materials and properties to calculate. Faced with this overabundance of opportunities, BIOVIA has made a consolidated effort to examine soft materials like polymers. Is it possible to take the material information from micro through meso to arrive at a Representative Volume Element (RVE) model? This approach is the simplest way to bridge the gap. Figure 1 represents the workflow from quantum mechanics, molecular mechanics and meso-scale. By understanding the atomistic scale, we can then connect to the continuum level to predict device-level properties. Machine Learning occurs at the initial stage. Here we want to find a new material based on experience or available information. We can move Machine Learning up or down in any of the methods based on data concentration.
A recent presentation at the SPE ACCE conference has adopted and tested this multiscale scenario over polyuria. The goal was to compare stress-strain curves at the micro and continuum level using Finite Element-RVE (FE-RVE). With an FE-RVE method, we can use FE tools to investigate and optimize the underlying constituent behaviors. Most importantly, FE-RVE captures the morphology of the microstructure with good accuracy. It also allows us to investigate “what-if” scenarios with the constituent behaviors.
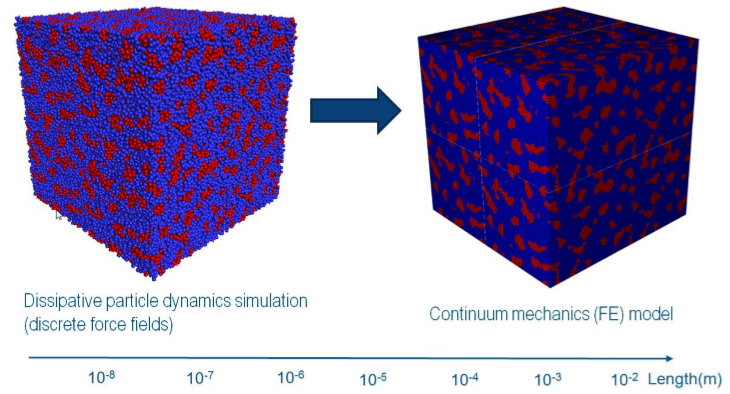
DPD Simulation with BIOVIA Materials Studio
Figure 2 shows how we can convert the morphology, as determined by Dissipative Particle Dynamics (DPD) simulation, into an FE-RVE model. To do this, we use the MESOCITE software within BIOVIA Materials Studio. With some appropriate periodic boundary conditions, we can subject the FE-RVE model to one or more types of loading. This can be uniaxial tension, simple compression, etc. Let us assume that we have high confidence in the soft and hard segment material properties. In this case, the RVE macro response ought to match some traditional macro test data.
The Challenge to Extrapolate
This first commercial trial in this field should be expandable to any materials of interest. The challenge remains to make the right force field at the micro and meso levels to produce the right structural model with an accuracy conducive to FE methods. The current method works with soft composite materials based on polymers and organic components. With the right force field, along with accurate and fast quantum mechanical methods, we should be able to extrapolate this method. For example, we want it to work with hard matrices like metals, metal oxides or a combination of both inorganic and organic matrices.
A Battery Example
The battery industry can leverage this methodology in battery design, from molecule to cell, module and system. Certainly, multiscale is key here, as chemistry affects the morphology of the electrode and its interfaces. We must investigate the micrometer scale to gain an understanding of the chemistry that occurs at the nanometer scale. Using ab initio-based force fields like COMPASS III we can generate material properties. Importantly, these can address key issues in cell design such as swelling of the anode in the macroscale models. We can start with reasonable accuracy and improve. Our ultimate goal is to understand the issue, fix it and improve production quality. This is especially important as we move to high-volume battery production for Electric vehicles (EVs).
Expanding Our Vision with a Different Perspective
The interesting thing about multi-scale is that we understand things better with a different perspective, as we move from the macroscale to the microscale. Moreover, the reverse is also true based on the prediction scale of the property. In the microscale, we rationalize properties such as diffusion and activation energy to predict the performance of the battery depending on the nature of the material. Consequently, we need to understand performance in the macroscale at the device level, whether it is a cell or a module pack in a battery. We understand changes in performance by studying the materials. For example, we understand activation energy at the microscale and swelling at the mesoscale. We use both properties to understand performance at the macroscale. Similarly, by passing information from micro to meso to macroscale, we expand our vision and better understand the full story.
Creating Seamless Connections within Macroscale Simulations
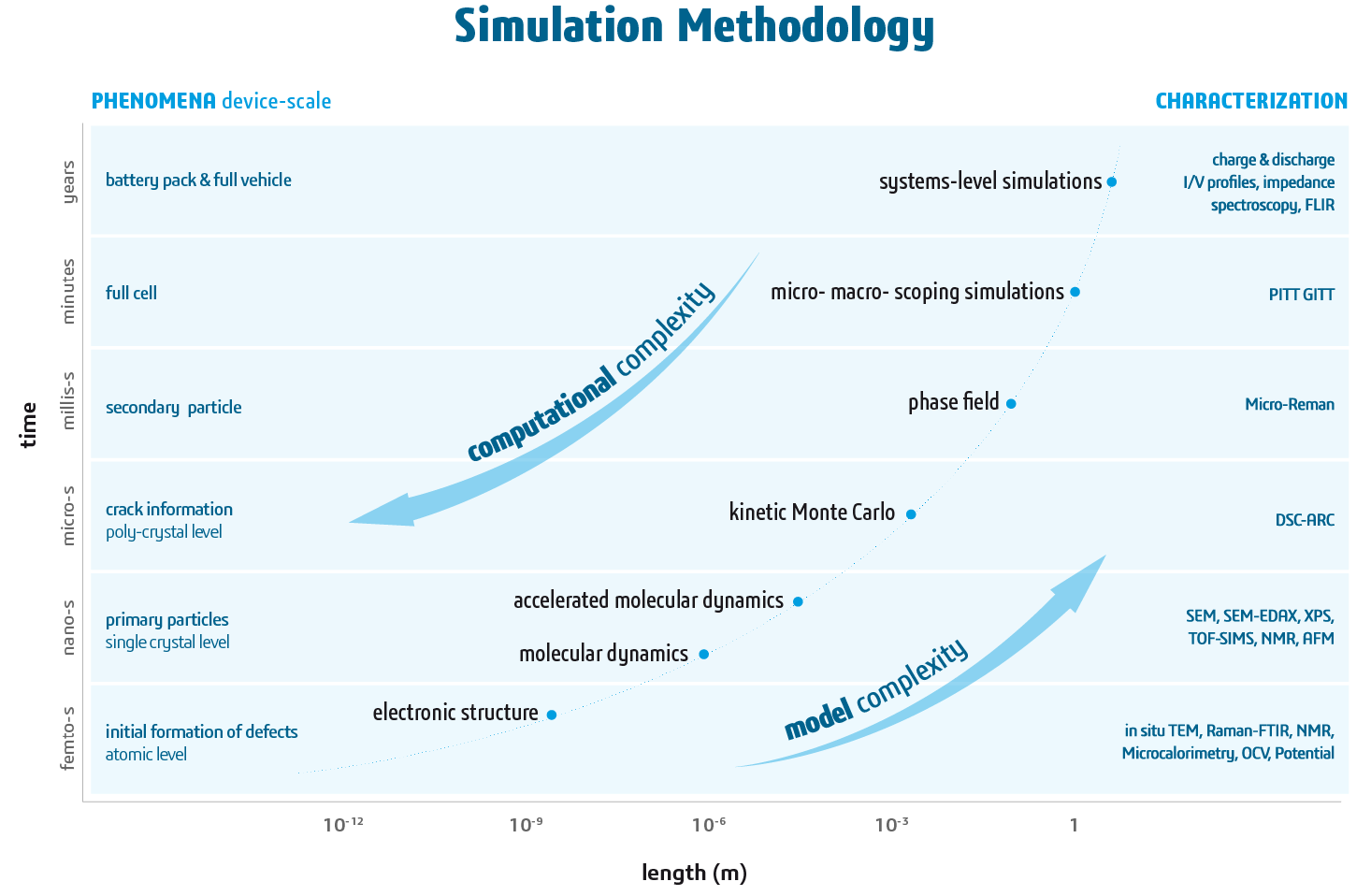
Figure 3 is a one-shot diagram. It shows how the predicted property maps to our current challenge. The challenge is to bridge the gap between molecular scale models and the continuum. To bridge this gap, BIOVIA is developing tools that focus on inorganic materials of interest such as, battery electrodes. One example of the available tools would be a Phase Field method.
The abovementioned tools bridge the gaps between the micro-meso-macro scales and create seamless connections within macroscale simulations. It advances “virtual twin” and experimentation cycle, increases product quality and shortens time-to-market.
Learn More about Materials Studio
References:
-
Multiscale simulations for material modeling, Victor Oancea, Tod Dalrymple, Huidi Ji, Jing Bi, Sakya Tripathy, James Wescott, Stephen Todd July 2016, Conference: SPE ACCE Conference.