Simulating Virtual Twins
Simulations are a cornerstone of a virtual twin approach to designing products and understanding scientific phenomena. A huge variety of predictive computational tools covers systems of all imaginable sizes. For example, recent computational studies related to the COVID-19 response range from atomistic simulations of viral proteins to computational fluid dynamics of entire hospitals.
A very simple example: thermally agitated water molecules simulated using BIOVIA Materials Studio.
As a general rule of thumb, simulations become more complex and detailed as we move from macroscopic to microscopic to nanoscale. In most cases, this also comes with increased computational cost for modeling a sample of a given size. For example, a system description of a simple lever spring could consist of a single equation with one variable (its position). It would be nearly impossible to model all the atoms simultaneously—even for the very small, spring-like cantilevers used in atomic force microscopes.
On the other hand, trying to understand the influence of a drug molecule on a protein receptor or the effect of chemical composition on the performance of a catalyst by simulating each molecule as a single particle would lack the chemical detail to be meaningful.
Choosing the right simulation method
Selecting an appropriate simulation method for any given problem is a highly nontrivial decision even for seasoned professionals, especially when it comes to mesoscopic and microscopic choices. This post attempts to provide a straight-forward rule to help you identify the correct simulation technique— specifically when you should make the switch from macroscopic (part-level) descriptions to particle-level calculations (atoms or beads), or when you should move from atomistic simulations to an electronic (quantum mechanical) representation.
The key to selecting the right computational method is to identify the underlying fluctuations driving the phenomenon you would like to understand.
What are the smallest changes without which the physical or chemical effect simply does not work?
Atomistic Simulations
In many situations, this identification is reasonably straightforward. For example, the thermal motion of atoms in any material gives rise to its entropy and to temperature-related properties such as heat capacity or thermal expansion. Understanding the origin of the underlying fluctuations at a nanoscale level typically requires atomistic simulations to study the motion of individual atoms with femtosecond resolution. This becomes slightly more difficult with metals where electrons can also carry a lot of the heat, suggesting that a quantum mechanical calculation would be required to treat the underlying electronic fluctuations. Alternatively, if you want to model the motion of heat in a material sample consisting of individual grains, then a larger scale, ‘representative volume element’ (RVE)-type of calculation would be appropriate. Of course, an all-atom simulation could take an inordinate amount of time without yielding much useful information.
Simulating the physical and chemical properties of composite materials is more complicated. Engineers are often interested in mechanical strength, weight and its statistical variation, which might be sufficient for part-level simulations. However, designing a material from scratch requires going back to the microscopic structure, e.g., studying fluctuations in atomic composition at the nanoscale. These fluctuations drive composite curing during manufacturing by determining which molecules cross-link and which ones remain unreacted. Hence, they play a defining role in establishing the material’s final properties. Understanding this process in detail requires atomic-level detailed simulations. You can then use the atomistic knowledge again in an RVE model to link to larger-scale thermal and mechanical properties that are interesting to engineers.
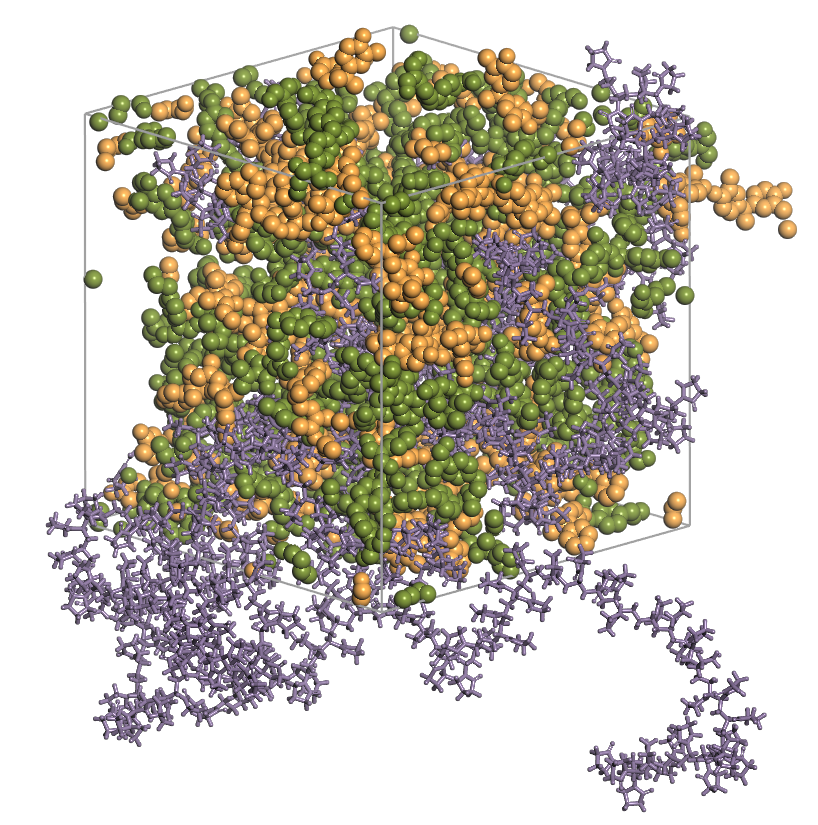
Simulating Degradation Processes
Batteries provide another Illustrative example, specifically their degradation mechanisms. Ultimately, the capacity fade found in phones and electric cars results from molecular-level fluctuations and gradual changes that occur on time scales ranging from hours to years. On the microscopic side, the degradation of individual components in electrolytes or the formation of lattice defects arise from molecular-level fluctuations requiring full atomistic or even quantum mechanical resolution. Some of these chemical degradations lead to the formation of chemically inactive layers storing lithium that build over many charge/discharge cycles. Here, system-level simulations covering the entire charge-discharge cycle are the only way forward. Another known degradation mechanism arises from the expansion and contraction of electrode particles (which is, of course, an atomistic process). The fluctuations that lead to the electrical disconnection of active material relate to micron-sized cracks. These are, in principle, comprehensible with finite element simulations.
Modeling Reaction Systems
Chemical reactions provide a final example for this discussion. At the smallest scale, these reactions result from thermal fluctuations causing atoms to explore their environment and ultimately cross a barrier into a new state. This motion from reactant to product is also governed by changes in the molecular orbitals. Understanding the rate of a given reaction in different environments requires highly accurate electronic structure simulations. However, moving beyond the single molecule reaction becomes seriously cumbersome with quantum mechanics. Moreover, the relevant fluctuations here relate to the local concentrations of reactants and products or their relative position on a catalyst surface. In this case, it is typically better to model reaction systems as a whole or to select a single molecular change as the smallest relevant ‘fluctuation’ and perform Monte Carlo-type simulations.
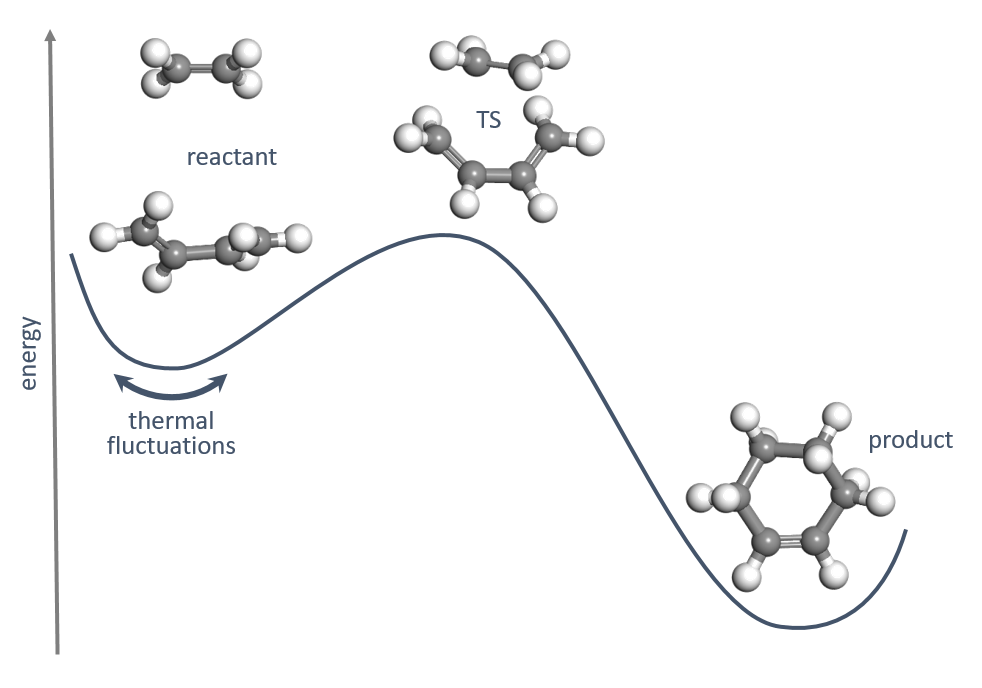
Dassault Systèmes software is capable of simulating all the material science challenges discussed in this blog. BIOVIA covers those aspects driven by microscopic thermal and electronic fluctuations. Our sister brands SIMULIA, CATIA and SOLIDWORKS provide world-class simulation software and expertise supporting larger-scale predictive simulations.